study
Technical Tiers: A New Classification Framework for Global AI Workforce Analysis
Authors
Programmes
Published by
Interface
April 29, 2025
Executive Summary
This study introduces a novel three-tiered classification system for global AI talent that addresses significant methodological limitations in existing workforce analyses, by distinguishing between different skill categories within the existing AI talent pool. By distinguishing between non-technical roles (Category 0), technical software development (Category 1), and advanced deep learning specialization (Category 2), our framework enables precise examination of AI workforce dynamics at a pivotal moment in global AI policy.
Through our analysis of a sample of 1.6 million individuals in the AI talent pool across 31 countries, we've uncovered clear patterns in technical talent distribution that significantly impact Europe's AI ambitions. Asian nations hold an advantage in specialized AI expertise, with South Korea (27%), Israel (23%), and Japan (20%) maintaining the highest proportions of Category 2 talent. Within Europe, Poland and Germany stand out as leaders in specialized AI talent. This may be connected to their initiatives to attract tech companies and investments in elite research institutions, though further research is needed to confirm these relationships.
Our data also reveals a shifting landscape of global talent flows. Research shows that countries employing points-based immigration systems attract 1.5 times more high-skilled migrants than those using demand-led approaches. This finding takes on new significance in light of recent geopolitical developments affecting scientific research globally. As restrictive policies and funding cuts create uncertainty for researchers in the United States, one of the big destinations for European AI talent, the way nations position their regulatory environments, scientific freedoms, and research infrastructure will increasingly determine their ability to attract and retain specialized AI talent.
The gender analysis in our study illuminates another dimension of competitive advantage. Contrary to the overall AI talent pool, EU countries lead in female representation in highly technical roles (Category 2), occupying seven of the top ten global rankings. Finland, Czechia, and Italy have the highest proportion of female representation in Category 2 roles globally (39%, 31%, and 28%, respectively). This gender diversity represents not merely a social achievement but a potential strategic asset in AI innovation, particularly as global coalitions increasingly emphasize the importance of diverse perspectives in AI development.
For European policymakers advancing ambitious initiatives like the €200 billion InvestAI program and AI Factories across 17 Member States, our research suggests that traditional conceptions of AI competitiveness focused solely on computational infrastructure or regulatory frameworks are insufficient. The composition, diversity, and mobility patterns of technical AI talent, particularly in the specialized Category 2, may ultimately determine which regions lead in the next phase of AI development.
As international talent flows respond to new pushes and pulls—from research funding environments to political attitudes toward scientific inquiry - regions that offer both technical resources and environments where researchers can pursue work freely across disciplines may find themselves with unprecedented opportunities to reshape the global AI landscape. Our findings indicate that Europe's distinct approach to AI development, balancing innovation with social values, positions it uniquely in this evolving talent marketplace.
This research contributes to a deeper understanding of global AI workforce dynamics at a moment when talent flows are poised for significant realignment, offering empirical foundations for more nuanced, evidence-informed policy formulation in an increasingly complex global landscape.
Introduction
AI technologies are reshaping our world, yet surprisingly little is known about the people creating these systems. While headlines trumpet the latest breakthroughs, we lack fundamental insights into the global AI workforce defined by the OECD as ‘workers possessing the skills necessary to develop and maintain AI systems’ – who they are, where they come from, and what drives their migration decisions. This knowledge gap isn't merely academic; it's a critical blind spot for countries competing in the global AI race.
While globalization has made it easier for goods and financial capital to move across borders efficiently, the mobility of human capital, particularly highly-skilled talent, has proven more resistant to this movement. This is made evident by the fact that only about 3% of the world’s population resides outside their country of birth. As Hein de Haas demonstrates in "How Migration Really Works," skilled migration follows distinct patterns shaped by both structural forces and individual agency – patterns that traditional policy frameworks often misunderstand.
Countries that successfully attract AI talent gain more than just technical workers; they secure a foothold in what may be this century's most transformative industry. Research reveals that nations employing points-based migration systems attract 1.5 times more high-skilled migrants than those using demand-led approaches. Yet the competition involves more than just entry policies. The most successful destinations combine streamlined immigration with robust integration supports, quality-of-life advantages, and environments where innovation thrives.
The challenge of understanding this workforce begins with definition and measurement. Traditional occupational classifications used in labour force surveys often lack the granularity necessary to capture the specialized skills required for AI development. AI Talent is often clubbed under a broader ICT talent category or remains under “high-skill” talent. This limitation has created a substantial gap in official statistics, making it difficult to answer fundamental questions about who is developing or directly utilizing AI in their professional roles, and to establish a common thread across different countries’ data. A software engineer might spend 80% of her time developing machine learning systems, while her colleague with the identical job title focuses exclusively on conventional programming.
Our paper addresses this gap by introducing a novel classification system for AI-related jobs. We distinguish between the different roles and skills within the AI talent pool to better understand AI talent ecosystems. Our classification system categorizes AI workers into three distinct levels: Category 0 comprises individuals in non-technical roles within the AI industry or those interested in AI but working in unrelated fields; Category 1 includes technical professionals such as software developers and data scientists who employ basic machine learning techniques but not advanced methods; Category 2 consists of specialists who directly develop or apply sophisticated deep learning techniques like neural networks, transformers, computer vision, and generative models. The results of our data analysis allow us to develop ideas on the following questions:
-
Which countries are developing technical AI talent, and which are primarily importing it?
-
What percentage of different national AI workforces consists of highly specialized technical talent?
-
Which nations are approaching gender parity in their AI workforce, and how have they achieved this?
The timing of this analysis is particularly urgent for Europe. As the EU launches an ambitious €20 billion public-private AI initiative and projects 21% growth in high-tech professions, the talent question looms large. Will Europe develop its own AI specialists, attract them from abroad, or watch them emigrate to competing innovation hubs? The answers will shape not just economic competitiveness but also the ethical development of AI systems that reflect European values.
By mapping the current landscape of AI talent migration and identifying effective talent attraction strategies, this paper aims to equip policymakers with the insights needed to position their countries advantageously in the global competition for AI expertise – a competition that will increasingly determine which nations lead in the digital economy.
Methodology
Data Sources
This study utilizes comprehensive workforce data provided by Revelio Labs, a workforce intelligence company that aggregates and structures publicly available professional profiles, job postings, and related sources. The dataset from 2024 encompasses 659 million individuals in the global workforce. From this extensive population, we identified approximately 1.6 million individuals who constitute the global technical AI workforce based on our classification framework.
The Revelio dataset includes gender estimates derived from census data on first-name gender distributions, enabling gender-based analyses of the AI talent landscape. Our analytical approach employs both absolute figures by country and per capita statistics, utilizing World Bank population data for national and metropolitan area comparisons.
Developing Technical Tiers in the AI Talent Pool
To systematically analyze the AI workforce, we developed a three-tiered classification system that categorizes individuals based on their level of involvement with AI technologies, technical expertise, and professional roles. Each category represents a distinct level of technical engagement with AI development and implementation.
Our previous data briefs on AI talent flows and distribution highlighted the limitations of existing talent classification frameworks. Through feedback and ongoing conversations with relevant stakeholders, including policymakers, industry representatives, and academic partners, we recognized a pressing need for a more nuanced understanding of the AI talent pool, particularly for policy development purposes.
Traditional binary classifications of AI talent have become increasingly outdated as the field has matured and diversified. These simplistic frameworks fail to capture the spectrum of AI-related roles that have emerged, from specialized deep learning researchers to applied data scientists and adjacent technical professionals who interact with AI systems. This limitation becomes particularly problematic when regions and nations attempt to assess their AI ecosystems, identify skills gaps, and develop targeted workforce strategies.
Our refined classification system offers direct insights into regional AI development trajectories and educational priorities. By distinguishing between regions where talent concentrates in fundamental AI research (Category 2) versus AI implementation and application (Category 1), policymakers can gain valuable perspective for economic development planning, immigration policies, and educational investments. This ecosystem understanding can also reveal opportunities for targeted upskilling initiatives. When demand for Category 2 AI professionals increases, organizations can efficiently upskill Category 1 professionals to address labor market gaps quickly. Similarly, targeted programs can help women transition from Categories 0 and 1 to Category 2, addressing gender disparities in technical AI roles.
-
Category 0: AI Curious and Literate individuals - Individuals not covered by the other two classes, such as those in non-technical roles or studying for degrees in unrelated fields. This class includes individuals who have an interest in deep learning, data science, and machine learning but do not currently work in a position or study in a field that directly involves these areas.
-
Category 1: Software & Data Professionals - Individuals working in technical roles or studying for degrees involving software development or data science, who may employ basic machine learning techniques in their work, such as linear regression. This class includes data scientists who do not work with advanced deep learning methods.
-
Category 2: AI Researchers & Engineers - Individuals currently employed in roles that directly involve developing, applying, or researching areas of AI deep learning techniques, such as computer vision, generative models, or other advanced machine learning applications. This class includes those who work with neural network architectures like self-attention transformers, RNNs, CNNs, LSTMs, etc.
Classification |
Category 0: |
Category 1: |
Category 2: |
---|---|---|---|
Educational Background Examples |
|
• Computer Science/Software Engineering degrees |
• Advanced degrees (MS/PhD) in Machine Learning, AI |
Technical Knowledge Examples |
• Basic understanding of AI concepts |
• Proficient in programming languages (Python, R, Java) |
• Expert-level understanding of neural network architectures |
Tools & Technologies Examples |
• Uses AI tools as end-users |
• SQL and database management |
• Advanced ML frameworks |
Job Roles Examples |
• Business Analyst |
• Software Developer |
• Machine Learning Engineer |
AI Problem-Solving Capabilities Examples |
• Can identify use cases for AI |
• Implements basic predictive models (regression, classification) |
• Designs complex neural architectures |
Projects Examples |
• Using AI tools to enhance productivity |
• Predictive analytics dashboards |
• Computer vision systems |
Approach
To address the challenges in distinguishing between our three AI talent categories, we employed a Large Language Model (LLM) classification approach. This methodology utilizes Natural Language Understanding capabilities to analyse professional profiles, educational backgrounds, and technical skills, offering an alternative to traditional rule-based classification systems.
We selected a stratified random sample of 1,000 profiles from each of 31 countries for our classification process. Our geographic coverage included all OECD nations plus India, limited to countries with more than 1,000 individuals in our initial AI talent pool. This selection builds upon previous research, which identified significant patterns in global AI talent distribution, including:
-
Significant migration of European AI talent to the United States
-
India's emergence as a primary source of AI talent globally
-
A pronounced divide between Northern/Western Europe (with higher proportions of international talent) and Southern/Eastern Europe
For a comprehensive background on previous AI talent research methodologies and findings, we refer readers to AI's Missing Link: The Gender Gap in the Talent Pool.
We selected Llama 3.1 70B as our classification model based on three criteria:
-
Alignment with our research transparency values through an open-source solution
-
Superior performance in preliminary benchmarking against proprietary alternatives
-
Enhanced data sovereignty, eliminating dependence on third-party APIs
Each individual profile was processed independently through the model using a specialized classification prompt optimized for our three-tiered taxonomy.
We approached this task through the lens of scientific NLP classification methodology. Our classification prompt was developed through systematic optimization using DSpy, a framework developed by the Stanford NLP group that enables rigorous, experimental approaches to prompt engineering. To validate our optimized prompt, we established a gold-standard test dataset comprising 100 manually classified profiles. Our final prompt achieved 80% accuracy on this test set, which falls well within the acceptable range for complex multi-class classification tasks using LLMs. This level of accuracy is comparable to or exceeds benchmarks established in similar classification studies, where accuracies for policy topic classification using LLMs ranged from 58-83%. Additionally, even studies implementing consensus mechanisms for similar complex classification tasks achieved only moderately higher accuracies of around 92%. It's worth noting that our classification task involves distinguishing between professional profiles that contain overlapping skills and ambiguous descriptions, making them inherently challenging to categorize even for human experts. Given these considerations, our 80% accuracy provides sufficient reliability for our large-scale classification task while acknowledging the inherent limitations of automated classification for nuanced professional categories.
Our classification methodology implements Chain-of-Thought (CoT) prompting, a state-of-the-art technique well-established in recent LLM research. This approach requires the model to articulate its reasoning process before providing a classification decision, which has been demonstrated to improve output quality and reliability. For each profile, the LLM provided:
-
A detailed rationale outlining the specific factors considered (skills, education, job titles)
-
A final classification label (0, 1, or 2) corresponding to our predefined categories
This approach not only improved classification accuracy but also provided valuable qualitative insights into the distinguishing characteristics of each talent category, enhancing both the reliability and interpretability of our classification results. The transparency of the reasoning chain further allows for systematic error analysis and potential refinement of classification criteria in future research.
Limitations
Sample Sizes
While we were able to obtain consistent samples of 1,000 workers for each country in our primary analysis, sample sizes varied significantly when classifying talent by country of bachelor's degree origin. This inconsistency in educational origin samples presents an important limitation to our study.
Countries with stronger data protection policies typically had fewer publicly available profiles, potentially resulting in less representative findings for these nations. Similarly, countries with smaller overall populations of technical workers contributed fewer samples to our dataset. These sampling inconsistencies should be considered when interpreting cross-country comparisons based on educational origin.
For transparency and to assist readers in evaluating the reliability of country-specific findings, a complete breakdown of sample sizes by country is provided in our last paper.
Using Undergraduate Degrees as Origin Proxies
The country of an individual’s undergraduate degree serves as a proxy for their origin, based on the assumption that most people pursue their early education in their country of origin. This method is generally reliable, as seen in these OECD data cases. Nonetheless, we acknowledge outliers like Australia and New Zealand, where the share of international undergraduates peaks at 28%, which could be due to English being their official language. Further evidence supports this hypothesis: the UK (18%) and Austria (17%) have high percentages of international undergraduates, contrasting with lower percentages in Southern European countries like Italy (4%) and Spain (2%). A case that challenges this hypothesis is the United States, where, despite the English language being the medium of education, only 4% of students at the undergraduate level are international students. For detailed statistics, please refer to the OECD report "Education at a Glance 2020." Not all countries of origin in our dataset are OECD countries. In our analysis, we verified a low percentage of international students from the most relevant countries. In India, for example, the percentage of international students at the undergraduate level is less than 0.5%.
When interpreting our findings, we take into account the variances in international student percentages across different countries. These variances can influence the perceived origin of AI talent. For example, our analysis may reveal that a percentage of AI professionals working in Ireland received their degrees in the UK. Given the UK's high proportion of international students (18%), a fraction of this group might actually originate from other countries. We believe that these effects do not substantially alter the primary insights regarding key source countries for AI talent.
Gender Prediction Limitation
While the data does not feature ground truth gender information, Revelio Labs estimates users' gender using census data information: each user's first name is checked against national census registries to estimate the gender shares of people with that name. This method can present issues with names that are not common: for those, the gender assignment is essentially random; nonetheless, they are, by distribution, a small minority of the population.
A significant limitation in our dataset is the binary representation of gender (male and female), which fails to capture the diverse spectrum of gender identities present in the workforce.
Given the scope and scale of our study, and the limitations of available global data sources, this binary representation is unavoidable for achieving consistent analysis across different regions and demographics. However, we recognize the importance of more inclusive gender representations and advocate for future improvements in data collection methods that can better capture the full spectrum of gender identities in the workforce.
Self-Reporting
The Revelio Labs dataset partially addresses the lack of self-reporting by including predicted skills. They also account for geographical differences in profile creation by applying sampling weights to adjust for roles and locations underrepresented in the sample (as described before). This approach helps approximate the true estimate of the underlying population as closely as possible.
This limitation is further mitigated in this paper's context, as we are mainly examining a more technologically advanced segment of the global workforce. These individuals typically possess the digital literacy and necessary equipment to create and maintain profiles on professional career websites. They often bear the benefits of self-reporting their skills.
Skill Taxonomy Limitations
The current skill taxonomy used by Revelio may not encompass all the necessary skills for specific AI domains, such as AI chip development. This gap means that professionals with specialized skills in areas like hardware or AI chip design might not be adequately recognized or included in the dataset. The absence of these specialized skills in the taxonomy can skew the understanding of the full spectrum of AI talent and hinder the comprehensive identification of expertise across all AI-related fields.
Findings
Chart 2 (a): Proportions of AI technical talent, classifications by country of employment
Chart 2 (b): Proportions of AI technical talent, classifications by country of origin
What you see:
The first chart displays the concentration of AI professionals across countries, measured as the number of workers per 1,000 people in each AI category. Countries are ranked based on their proportion of category-2 AI professionals, from highest to lowest. This visualization helps compare how different nations are currently employing AI talent across various specializations relative to their population size.
The second chart presents the distribution of AI professionals based on their country of origin, defined as where they completed their bachelor's degree. Countries are again ranked by their proportion of category-2 AI professionals, from highest to lowest. This visualization reveals which nations are producing AI talent in different categories and allows for comparison between where AI professionals are trained versus where they currently work.
What it means:
Of the countries examined by this paper, South Korea has the highest proportion of Category 2 roles, making up 27% of its AI labour force, followed by Israel and Japan, at 23% and 20%, respectively. The highest EU ranking countries are Poland and Germany, in fourth and fifth place respectively. This concentration of highly skilled AI experts could be because of different strategic policies aimed at ensuring global leadership positions in AI through the attraction of top talent.
Korea has key strategic government initiatives to bring a top-tier AI research hub to Korea to ensure competitiveness in the field of AI, as well as expand digital capacity domestically through investments in digital education to ensure a global leading role.
Within Europe, Poland and Germany’s leading roles could be attributed to both strong industrial economies, as well as strategic policies to attract companies and talent. Poland, ranked fourth in Category 2 roles, which could be explained by the implementation of its national strategy for AI, which focused on both AI company growth and innovation, and investing in domestic capacity building. Poland is one of the main destinations for Ukrainians fleeing the war, mainly women and children, with refugee numbers stabilized at around one million and a 65% employment rate - the highest among countries accepting significant Ukrainian refugees. Demand for foreign talent continues to grow as Poland drafts a new digitization strategy and invests in new institutions to foster collaboration with the European AI ecosystem.
Germany, ranked fifth, boasts a large concentration of elite institutions that are some of the largest publishers of both fundamental and applied research in the global ecosystem, creating a strong skills environment that may lead to its strong positioning as both an origin and destination of top global technical talent. Countries like Switzerland, with a strong focus on attracting foreign talent, rank highly and remain competitive through deliberate efforts to attract key AI talent.
The EU’s AI workforce has a higher proportion of technical experts in Category 2 talent compared to leading AI nations like the US and UK. Many of the European national AI strategies lay out goals to increase the attractiveness of domestic AI industries, including through the building up of institutions and attracting top talent, and the EU maintains a competitive advantage of having more AI experts per capita than the US or China.
Countries with more mature tech industries may have simultaneously diversified AI workforces to include additional non-technical roles, like product management, strategic operations, or sales, which do not directly contribute to the building of AI systems. Additionally, tougher political climates led to higher scrutiny of many international AI workers in the US may also be a limiting factor in its ability to attract and retain top talent out of countries it views as geopolitically sensitive, like China. Despite the EU’s technical advantage, a significant amount of its AI talent from the Global South still faces high attrition rates in favour of the US. However, many leaders in Europe see the current American political climate as an opportunity for the region to attract disillusioned top talent from the US. AI engineers, entrepreneurs, and researchers may see Europe as an attractive alternative location with more freedom and commitment to human-centric development of technology, reversing this migration trend in the coming years.
Chart 3(a): Proportions of international AI technical talent, classification by country of origin
Chart 3 (b): Proportions of international AI technical talent, classification by country of destination
What you see:
The first chart displays the distribution of international AI technical talent based on their countries of origin, showing the proportion of professionals in each AI category per 1,000 people who have migrated from their home countries. Countries are ranked from highest to lowest based on their proportion of category-2 talent production. For example, 32% of the Swiss talent outside Switzerland falls in Category 2.
The second chart illustrates the same metrics but classified by destination countries, revealing where international AI professionals are currently employed. For example, 27% of the non-Korean AI talent in South Korea falls in category 2.
This comparison helps visualize both which countries are producing AI talent that moves abroad, and which countries are most successful at attracting international AI professionals across different categories.
What it means:
The purpose of these graphs is to better understand the relationship between domestic talent development and international recruitment, capturing nuances of which countries' talent may originate from, but later be lost to other countries, and which countries attract a lot of international talent. These charts highlight the complexity hidden within AI workforces globally, as countries seek to balance the flow of skilled labourers. Although typically considered global AI leaders, the US and the UK have a lower proportion of Category 2 roles, even as a destination country for top talent. More stringent immigration requirements may introduce challenges in attracting foreign professionals, especially those with non-traditional AI backgrounds. A 2024 Stack Overflow survey of 65,000 contributors found that only 66% of developers have either a bachelor's or master's degree, with less than half studying coding formally, and formal education requirements may result in missing out on thousands of AI professionals.
The European AI industry demonstrates a dual focus on domestic talent development and international recruitment. Countries like Poland, Switzerland, and Germany represent both talent origin countries and destinations. Previous research indicates that the high levels of AI talent in those countries may be due to factors such as more liberal migration policies to facilitate the hiring of top talent, like Germany's Blue CardEU or Poland’s new international worker protections. Germany's emphasis on international talent is further evidenced by recent research showing professionals with limited German language competency earn higher median salaries (79.8k) than those with high fluency (67.3k). Recognizing the enormous labour shortage of skilled workers, Germany introduced digitalisation and modernisation of consular services to enable a more streamlined and easy digital process to obtain a visa, preventing large bureaucratic obstacles from dissuading top talent from applying to work in the country.
Other factors driving the AI lead in Central and Eastern Europe may include extensive investment to expand infrastructure, strengthened VC environments, and strategic focus on the concentration of skilled technical workforce. Lower-ranking destination countries are also aiming to attract top professionals: Italy laid out ambitious plans to recruit international talent while boosting local capacity in AI.
Countries that utilize supply-side policies, like points-based systems (PBS), attract nearly 1.5 times the number of high-skilled workers than countries utilizing other immigration policies. Canada, Australia, and Japan developed more flexible recruitment approaches, in part due to their lower rankings as talent origin countries. These immigration systems garnered positive perceptions among international professionals, improving the systems’ ability to attract global AI talent.
English-speaking nations maintain an advantage in international talent recruitment, with lower linguistic barriers to entry for many Anglophone origin countries. However, non-Anglophone countries adapted their immigration policies to balance the playing field: Austria’s PBS rewards English proficiency, reflecting an increasing international business focus.
By contrast, there are many countries that are large sources of international talent that fall much lower in the ranks as destination countries. India does not have extensive amounts of professionals in Category 2, coming in at the bottom of the countries examined for this paper. However, it does relatively better as an origin country for international AI expertise. Russia, Iran, and China may have lower recruitment of international talent due to a highly qualified domestic talent pool. China alone represents nearly half of the world’s AI experts. They may also struggle to attract talent due to political instability and economically disadvantaged zones like Russia, which is grappling with the impacts of sanctions imposed after the invasion of Ukraine. Other reasons could be language barriers.
Chart 4: Source Distribution of Category 2 AI Professionals: Domestic vs. Foreign
What you see:
This visualization illustrates the composition of Category 2 AI jobs across countries, comparing domestic and international talent. Each data point represents a country positioned according to its proportion of non-migrant professionals (x-axis) versus migrant professionals (y-axis). The plot reveals which nations rely more heavily on homegrown talent versus those that attract significant international expertise in Category 2 AI roles, highlighting global talent distribution patterns and potential correlations between domestic capacity and international attraction.
What it means:
Most countries examined in this paper have relatively close proportions of migrant workers and domestic workers in Category 2 roles. This indicates that among the countries examined in this paper, few have majority domestic AI talent pools, and the proportions are not drastically different. Countries with a competitive edge in AI, like the US, Ireland, and the UK, all have a slightly higher proportion of migrant AI talent. Countries like Japan and the US, with higher proportions of migrant labour, also offer higher wages, creating attractive conditions for highly skilled international workers.
South Korea, Japan, and the US have competitive advantages for top AI talent and have immigration policies and favourable financial incentives to attract and retain AI professionals across different sectors.
Many European countries with higher proportions of foreign AI talent have more favourable immigration policies or offer benefits to attract skilled workers. Czechia recently loosened restrictions around foreign workers from top AI origin countries like South Korea, Canada, Israel, and New Zealand to work without needing a specific permit (Refer to Figure 1), while Finland relaxed immigration requirements for skilled workers through a talent boosting program and offers programs inviting international professionals to explore the country for potential business opportunities. The Netherlands offers an “expat” tax deduction scheme to create additional financial incentives for foreign talent, a trend also seen in Spain and Portugal. These countries enjoy the benefits of attracting more foreign talent and building more robust AI industries than EU countries that fail to implement attractive policies.
Countries failing to enact policies that make them desirable destinations might suffer from further AI talent shortages. Greece, which ranks the lowest of the EU countries with a proportion of Category 2 both domestic and international talent, may be causing its own brain drain due to a lack of a national AI strategy. It has some of the highest numbers of hours worked per year in OECD countries, and introduced a six-day workweek.
Chart 5: Proportions of Males and Females classified as category 2
What you see:
The chart shows the proportion of category 2 professionals for males and females, divided by country. Compared with Charts 1 and 2, where we examine the proportion of jobs classified from level 0 to 2, this chart focuses on the gender breakdown of the most technical AI talent of different national workforces. Countries are ranked from highest to lowest proportion of female workforce in Category 2 roles.
What it means:
Contrary to the overall proportions of highly technical AI professionals, the EU leads in female representation in technical roles, occupying seven out of the top ten rankings. Finland, Czechia, and Italy have the highest proportion of female representation globally. This does not mean gender parity has been achieved; rather, it echoes findings of previous interface reports, no EU country selected for this study has been able to ensure parity in the representation of women in the AI talent pool. Although women accounted for 32.8% of total graduates in STEM fields in the EU in 2021, those percentages have not yet translated to higher rates of participation in the labour market, especially in more senior positions.
Finland leads overall with the highest proportion of female AI talent in Category 2, representing 39% of the total labour force. While this could be driven by a relatively small sample size of Finnish AI labour market conditions, it could also reflect strong gender equality policies like the Act on Equality between Women and Men, which requires universities and employers to address gender gaps in things like salaries and working conditions. The country also boasts a strong work-life balance, including a historic flexible working schedule offered by a majority of companies since 2011. Now, it is mandated by law that employees in Finland are offered flexible working hours and locations for at least half of their working hours, and employees can also bank their working hours by working longer at other periods, enabling professionals to meet personal commitments without it coming at the expense of their professional careers. This may be a contributing factor to the rates of gender parity in Category 2 roles, but direct causality cannot be attributed.
Similarly, Czechia, with 31% of Category 2 talent held by women, has observed a steady increase in women applying to study AI at a tertiary level in the country and strategically monitors R&D for gender considerations. Czechia has strategically aimed to increase the representation of women in research, including through equalizing opportunities for women and men and acknowledging the barriers to women building careers in the field, due to things like prejudice or the demands of parenthood.
With a workforce of 28% women, Italy similarly boasts not only strong parental leave policies and good work-life balance but also has the highest percentage of AI papers published by at least one female author among OECD countries.
Our data indicates that destination countries for top AI talent tend to have lower proportions of female representation in Category 2 roles. Both the US and Korea only see women in 14% of technical roles, and Japan has the lowest proportion of women in Category 2 roles at 11%. Despite efforts to introduce quotas at universities, stigmas around female STEM graduates allegedly being unable to find husbands or start families persist. Further compounding these stereotypes are systemic barriers to studying, that keep women out of STEM careers. Contrast that with top AI talent origin countries, where India has 22 % women in Category 2 roles. This number reflects a growth that has been part of a deliberate set of policies to increase female participation in the Indian labour force and invest heavily in AI education. While there is relatively high participation from females in highly technical roles in India, Indian females are very underrepresented in AI roles outside India. This could hint at disincentives for females leaving their home country to pursue professional opportunities.
Chart 6: Male-Female proportions of Senior Executive AI talent
What you see:
The chart shows the proportion of each gender in senior executive AI roles, ordered from the highest to lowest proportion of female workforce in senior executive AI roles. The female share is in blue. We have defined the seniority based on Revelio Lab’s existing seniority metric.
What it means:
The graph illustrates ‘the leaky pipeline,’ a phenomenon in which women are progressively missing from more senior roles in STEM fields. Previous interface research similarly found a diminishing representation at higher seniority levels in the AI industry, and this data indicates that the disparity has not been sufficiently addressed in any of the countries examined by this paper.
Similar to the previous figure, Finland leads the overall proportion of women in senior executive roles at 41%, followed by Lithuania (33%) and Iceland (26%). Italy, which ranked third in proportion of female workers in Category 2 roles, drops to seventh place with only 16% of senior roles occupied by women. Many countries examined in this paper have less than a fifth of senior executive roles held by women. This disparity is probably driven first by cultural norms and gender stereotypes, starting first with education, weakening girls’ participation in STEM subjects, and later by gender discrimination and unconscious biases in hiring practices, promotion pathways, and even funding opportunities, which can hamper a woman’s career progressions. Additionally, women face challenges like the gender pay gap and slower progression to senior roles, as they may leave to take career breaks or reduce their working hours, creating additional obstacles to career advancement.
If the EU seeks to meet its commitments to increase female participation in AI by "the means of promotion of equitable access and participation in the digital sphere and the empowerment of individuals to identify, mitigate, prevent and eradicate gender biases, stereotypes, discriminations, and violence,” it must continuously monitor the proportions of gender in its AI workforce. Our data indicates significant gender imbalances at every level of seniority and skillset within the EU. European policymakers have recognized this, calling for ‘diversity of the development teams, including gender balance,’ in the AI Act, but recognizing the urgency of this work has not yet led to ensuring meaningful and consistent representation of marginalized groups like women.
Conclusion
The application of our three-tiered classification system to global AI talent has yielded several new insights with significant implications for research, policy, and practice. By disaggregating the AI workforce into non-technical talent (Category 0), technical implementation specialists (Category 1), and deep learning experts (Category 2), we have uncovered patterns in talent distribution that challenge conventional narratives about global AI competitiveness.
A central finding of this research is the complex relationship between national innovation systems and specialized talent development. While the proportion of Category 2 talent broadly correlates with nations' overall AI investments, notable exceptions emerge. Poland's strong performance despite more modest overall AI spending suggests that targeted educational policies can yield disproportionate returns in developing specialized expertise. Conversely, countries with substantial general AI investment but lower proportions of Category 2 talent may be encountering structural barriers that warrant further investigation.
The analysis of international talent flows reveals an unexpected directionality in migration patterns. Origin countries with the highest proportions of Category 2 talent among their diaspora (Switzerland 32%, Korea 27%, Russia 25%) demonstrate that expertise development and retention are distinct challenges requiring different policy approaches. Most countries examined in our study show equal or higher proportions of Category 2 talent among migrant workers compared to domestic talent. This consistent pattern suggests a global marketplace that rewards specialized expertise with enhanced mobility.
Our examination of gender representation contributes empirical evidence to ongoing discussions about diversity in AI development. The finding that female representation in Category 2 roles averages only 22% globally confirms persistent gender disparities, but the significant variations between countries, from Finland's 39% to Japan's 11%, indicate that these disparities are not inevitable but are shaped by specific policy environments and workplace cultures. The stark drop-off in female representation at senior executive levels across all countries points to a universal challenge requiring targeted interventions.
Methodologically, this study demonstrates the value of developing more nuanced classification systems for analysing technical workforces. Standard occupational classifications and binary distinctions between "AI" and "non-AI" workers have proven insufficient for capturing the heterogeneity within the field.
As nations increasingly recognize talent as a critical dimension of AI leadership, the framework and findings presented in this study offer a more sophisticated foundation for policy development. Effective strategies must address not only the aggregate size of the AI workforce but its composition across the three tiers we have identified, with particular attention to the movement between categories through targeted interventions. The nations that effectively navigate these complex talent dynamics, balancing domestic expertise cultivation with strategic international recruitment and addressing systemic diversity challenges, will not only strengthen their digital sovereignty but ultimately shape the responsible, human-centric AI ecosystem that defines Europe's distinct path in the global AI landscape.
Acknowledgements
This research was generously supported by the Carl Zeiss Stiftung as part of the project 'Strength and weaknesses of the German and European AI ecosystem - talent in the focus.'
We extend our sincere gratitude to our colleagues at interface: Sabrina Santos Peres and Alba Peschke Montiel for their invaluable research assistance; Luisa Seeling for her meticulous editorial work; Alina Siebert for her expertise in formatting and creative visual designs; and Nicole Lemke for her thoughtful feedback on early drafts. Special thanks to Ernesto Oyarbide-Magaña and Sebastian Rieger for their efforts in disseminating this work. We are also grateful to the anonymous reviewers whose insightful comments significantly improved this manuscript.
We would also like to express our appreciation to the numerous scholars, practitioners, and policy experts who engaged with our previous research and whose thoughtful questions and feedback encouraged us to develop this more comprehensive study. Your intellectual curiosity and support have been instrumental in shaping this work.
This paper is being published as part of the project 'Strength and weaknesses of the German and European AI ecosystem - talent in the focus'. The project is funded by the Carl Zeiss Stiftung.
Table of Contents
-
-
Chart 2 (a): Proportions of AI technical talent, classifications by country of employment
-
Chart 2 (b): Proportions of AI technical talent, classifications by country of origin
-
Chart 3(a): Proportions of international AI technical talent, classification by country of origin
-
Chart 4: Source Distribution of Category 2 AI Professionals: Domestic vs. Foreign
-
Chart 5: Proportions of Males and Females classified as category 2
-
Chart 6: Male-Female proportions of Senior Executive AI talent
-
Authors
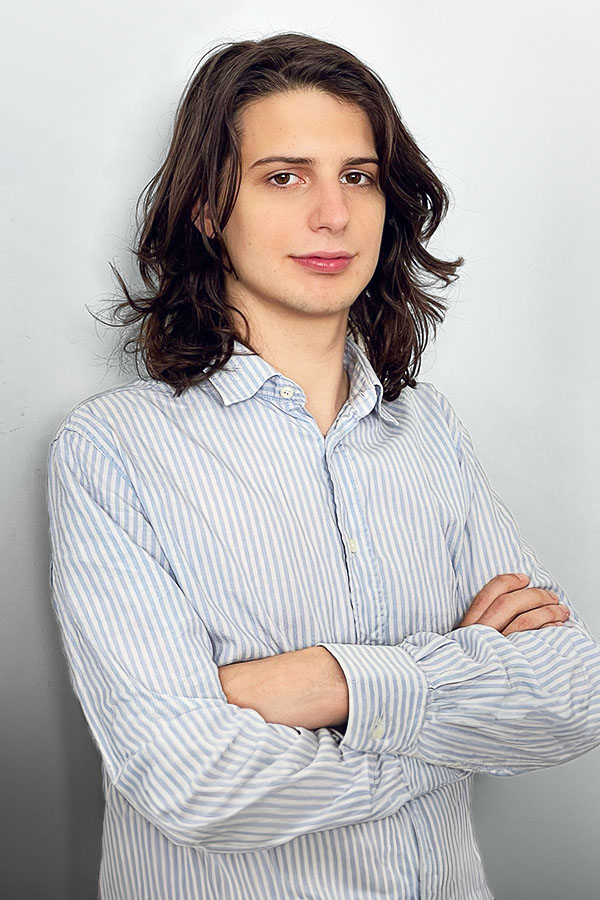
Ruggero Marino Lazzaroni
Data Scientist
Related Content
Data Brief
Where is Europe's AI workforce coming from?
Immigration, Emigration & Transborder Movement of AI talent
Siddhi Pal
July 31, 2024
Position Statement
The Talent Domain: Addressing Workforce Challenges in the EU's AI Continent Action Plan
Siddhi Pal
April 9, 2025
Data Brief
AI's Missing Link: The Gender Gap in the Talent Pool
Siddhi Pal, Ruggero Marino Lazzaroni, Paula Mendoza
October 10, 2024