data-brief
AI's Missing Link: The Gender Gap in the Talent Pool
Authors
Programmes
Published by
Interface
October 10, 2024
Executive Summary
The artificial intelligence (AI) industry is facing a critical diversity crisis, with women severely underrepresented across all seniority levels. This data brief quantifies the multifaceted underrepresentation of women in the global and European Union (EU) AI talent pool, highlighting the pressing need for targeted interventions to increase female participation in this rapidly expanding field.
Our analysis of data on nearly 1.6 million AI professionals worldwide reveals stark gender imbalances. Women comprise only 22% of AI talent globally, with even lower representation at senior levels – occupying less than 14% of senior executive roles in AI. Within the EU, the disparity is equally concerning. Europe has closed 75% of its gender gap 1 , with Sweden and Germany among the top five European economies in closing the gender gap. However, our data reveals a stark contrast in the AI sector: Germany and Sweden have some of the lowest female representations in their AI workforces in the EU, at 20.3% and 22.4% respectively. This discrepancy raises serious questions about the unique barriers faced by women in the AI field.
This gender gap in AI talent pools is significantly more pronounced than in the overall workforce. In countries like Portugal and Estonia, which have achieved near gender equity in their general labor force, the AI sector shows dramatic imbalances of up to 51%. Even in leading AI hubs, diversity remains a challenge. In Frankfurt, a major European tech center, just 19% of AI talent is female. Milan leads among European AI hubs with 30.7% female AI professionals, still far from parity. This underrepresentation of women in AI has serious implications, potentially exacerbating biases in AI systems and limiting innovation 2 3 4 . While having more women and underrepresented groups in AI won't automatically fix existing biased data sets, it will drive a push for more diverse and representative data collection over time. Diverse teams are more likely to question the status quo, recognize gaps in data collection, and advocate for more inclusive practices.
The gender gap also represents a significant loss of potential talent in one of the most crucial industries of our time. As AI becomes increasingly central to innovation and economic growth, we're essentially operating at partial capacity. In our last data brief, we highlighted the heated global demand for AI talent and the brain drain causing a significant talent shortage in many countries. Increasing the number of women in AI is not just about equity—it's about meeting the pressing need for more AI specialists overall.
Addressing this diversity crisis is not just a matter of equality, but crucial for developing AI systems that work equitably for all of society. As the EU aims to become a global leader in AI, closing the gender gap must be a priority. This will require multi-faceted efforts, from encouraging more girls and women to pursue STEM education to improving workplace cultures and retention of female talent in AI careers. With AI poised to reshape numerous industries, ensuring diverse perspectives in its development is essential for realizing the full potential of this transformative technology and upholding democratic values in an increasingly AI-mediated world.
This paper is being published as part of the project 'Strength and weaknesses of the German and European AI ecosystem - talent in the focus'. The project is funded by the Carl Zeiss Stiftung.
Introduction
As artificial intelligence (AI) systems become increasingly integrated into our daily lives, the question of who develops and operates these technologies becomes critical. Previous research has established that the AI industry faces a significant diversity crisis, particularly in terms of gender representation 5 6 7 8 .
This study provides further evidence to strengthen this argument, offering quantitative data on the multifaceted underrepresentation of women in the global and European Union AI talent pool. To establish a comprehensive foundation for our diversity analysis, we first present a broad overview of the AI talent landscape, featuring data visualizations on global talent distribution and concentration.
The importance of diversity in AI development cannot be overstated, as AI systems are not neutral; they reflect the biases, perspectives, and values of their creators. The homogeneity of the AI workforce has direct consequences on the technologies being developed, often reflecting and amplifying existing societal biases related to gender and race. The implications of this gender gap extend far beyond the AI industry itself. As AI systems increasingly mediate our interactions with institutions, services, and each other, the lack of diverse perspectives in their development raises serious concerns about fairness, bias, and representation.
The concept of "exclusion overhead," introduced by Joy Buolamwini 9 , is particularly relevant here. It describes the additional burden individuals must bear when technological systems are not designed with their needs or characteristics in mind. For instance, an AI-powered facial recognition system developed primarily by male professionals may perform poorly in recognizing female faces, especially from diverse ethnic backgrounds. This creates an exclusion overhead for women and minorities, who may face more frequent misidentifications or system failures, leading to practical challenges in areas like security checks or device access.
Moreover, the gender gap in AI represents a significant loss of talent and potential innovation in a field that is crucial for future economic growth and technological advancement. By increasing diversity in AI development teams, we can not only reduce exclusion overhead but also enhance the overall effectiveness and applicability of AI systems across diverse user groups. While the arguments for closing the gender gap in AI based on representation, inclusion, and democratic values are paramount, there is also a compelling pragmatic case for increasing female participation in the AI workforce. The rapidly growing field of AI faces a significant talent shortage, and increasing the number of women in AI is not just about equity—it's about meeting the pressing need for more AI specialists overall.
Our analysis draws from a robust dataset of nearly 1.6 million AI professionals worldwide, providing a comprehensive view of the gender distribution in the AI workforce. We examine this data through various lenses, including geographical distribution, seniority levels, and comparisons with overall workforce trends. This multifaceted approach allows us to identify key areas of concern and potential intervention points.
The global AI landscape reveals significant disparities in gender representation across different regions, zooming into the European Union, we get the full and complex picture in this paper. The EU's ambition to become a global leader in AI development and deployment adds urgency to addressing the gender gap in this field. A particularly concerning trend emerges when comparing the gender balance in AI to that of the overall workforce. In many countries, including those that have achieved near parity in their general labor force, the AI sector shows dramatically larger gender imbalances. This suggests that the AI industry faces unique challenges in attracting and retaining female talent, requiring targeted strategies to address these specific barriers.
This data brief aims to provide policymakers, industry leaders, and educators with the insights needed to develop effective strategies for closing the gender gap in AI. By presenting a detailed analysis of the current state of gender representation in AI, we hope to catalyze action towards creating a more diverse and inclusive AI workforce, ultimately leading to more equitable and efficient technological solutions that benefit all of society.
Data
We define AI talent based on a combination of skills and job roles. Revelio Labs, a workforce intelligence company, provided us with recent (2024) individual-level data on 659 million people in the global workforce based on an aggregation of publicly available professional profiles, job postings, and other sources. We use our own definition of AI talent based on skills and job titles to filter out close to 1.6 million people (almost twice the population of Brussels) forming the global AI workforce.
Revelio's dataset incorporates gender estimates for individual users, derived from census data on gender distributions associated with first names. Our analyses employ both absolute numbers by country and per capita statistics. For the latter, we utilize World Bank population data as a reference, encompassing both national and metropolitan area figures.
You can find the details of our approach, the definitions used by Revelio and by us, and also some considerations on possible limitations to the validity of the data in our Methodology section.
Findings
Chart 1: AI talent density by country
What you see
The first chart presents the concentration of AI talent per capita across various EU and Non-EU countries, calculated as the number of AI professionals per 1,000 people. The second chart shows the absolute number of AI talent across countries.
What it means
Singapore leads the nations studied in this paper with the highest concentration of AI talent per capita, closely followed by Luxembourg, which tops the EU rankings. The Netherlands, while second in AI talent density within the EU, secures the sixth position globally. This data illustrates the diverse distribution of AI expertise across both EU and non-EU countries, highlighting the global competition for AI talent. Notably, several EU member states surpass the United States in AI talent concentration, reflecting Europe's strong position in boasting a significant AI talent pool.
The first South-Asian country with a national AI strategy 10 , Singapore is making bold moves to become a global AI powerhouse, aiming to triple its AI expert workforce to 15,000 as part of its second National AI Strategy (NAIS 2.0) 11 . This ambitious plan builds upon the success of the AI Apprenticeship Programme, which has already graduated 300 Singaporeans as of September 2023. The strategy encompasses targeted AI training programs, intensified AI adoption across enterprises, and a welcoming approach to global AI talent. A key feature is the establishment of, what they call, an "iconic" site to nurture the AI community, fostering innovation and collaboration. 12 13 The government is also launching an AI Accelerated Masters Program in partnership with local universities. This initiative will be complemented by more rigorous AI practitioner training for students, including scholarships and international internship opportunities in AI-focused roles. 14
The second smallest country in the EU by population, Luxembourg has the highest concentration of AI talent per capita on the continent. The country relies heavily on a foreign workforce (74%) 15 , and particularly for AI talent, it relies almost entirely (95%) on individuals with foreign degrees, which is a strong indicator that this group indeed consists of mostly foreign nationals. 16 . It's important to note that this trend is influenced by Luxembourg's unique demographic composition, with foreign nationals comprising almost 50% of its population.
Attracting and retaining foreign talent has long been essential for Luxembourg, prompting the implementation of various policies and strategies to achieve this goal. Notable examples include the Roadmap for the Development of a National Talent Attraction, Development and Retention Strategy (2022), the Recovery and Resilience Plan of Luxembourg (2021), and the Digital Skills and Jobs Coalition (2017) 17 . The latter two initiatives outline specific measures to enhance digital skills in Luxembourg. In 2020, Luxembourg unveiled its strategic vision for AI 18 , backed by substantial government funding. This strategy encompasses key actions to cultivate AI skills and expertise, including investments in upskilling programs, lifelong learning initiatives, and specialized digital and AI-related training. Furthermore, the country's approach focuses on retaining AI talent within Europe and encouraging more women to pursue AI studies.
These two cases demonstrate how countries can effectively maintain and expand their AI workforce through targeted strategies and policies, emphasizing the importance of comprehensive approaches to attracting, nurturing, and retaining global talent in the AI sector.
Chart 2: Leading metro hubs for AI expertise
What you see
These two charts illustrate the leading global city hubs for AI talent. The left chart ranks cities based on AI talent per capita, while the right chart ranks them according to the absolute number of AI professionals. Both charts showcase the top 25 cities in their respective categories.
What it means
The United States dominates the landscape of AI talent hubs, with San Jose, San Francisco, and Seattle claiming the top three positions globally. San Jose leads the pack with an impressive ratio of nearly 25 AI professionals per 1,000 workers. San Francisco and Seattle follow, boasting 11 and 10 AI specialists per 1,000 workers, respectively. In contrast, the European Union's presence in the top 25 hubs is limited to just three cities. Amsterdam stands out as the EU's premier AI hub, ranking fourth globally, while Berlin secures the ninth spot worldwide and second within the EU. Interestingly, Paris, which leads the EU in absolute numbers of AI talent, ranks third in the EU and 12th globally when adjusted for population size.
The United States has emerged as a formidable competitor for AI talent, and has taken a comprehensive approach to attracting, retaining, and developing AI professionals across various sectors.
In late 2023, the Biden-Harris Administration issued a landmark Executive Order to ensure U.S. leadership in harnessing AI's potential while managing its risks. This order mandated increased investment in AI innovation and new initiatives to attract and retain AI expertise. Key provisions include streamlining visa processes for non-citizens working on or studying AI and related technologies, reviewing and modifying immigration policies to expedite pathways for AI experts, establishing a program to identify and attract top AI talent, and creating a Task Force to accelerate and monitor the hiring of AI talent across the Federal Government 19 .
San Jose, situated in the heart of Silicon Valley, exemplifies this leadership in AI innovation and talent cultivation. The city's unique position stems from housing world-leading tech companies and a robust venture capital ecosystem. Its proximity to prestigious institutions like Stanford and UC Berkeley facilitates collaboration and innovation. The symbiotic relationship between academia and industry creates a dynamic environment for talent development, with students and researchers frequently transitioning to roles in high-tech companies and startups 20 .
The San Jose City Council has taken proactive steps in adopting AI technologies and policies. These include launching the GovAI Coalition to develop guidance for public sector AI implementation, implementing AI-based solutions for public services, and approving the city's first budget allocation to advance AI use in improving city services. This approach not only enhances efficiency but also serves as a model for how local governments can leverage AI to improve services and attract top talent. 21
Amsterdam has established itself as one of Europe's strongest AI ecosystems and a leading magnet for AI talent. The city's success is built on a robust foundation of industry leadership, academic collaboration, and government support. Numerous international companies have established AI research labs in Amsterdam, while several AI-oriented multinationals have chosen to locate their headquarters there. This corporate presence is complemented by a thriving community of small and medium-sized AI businesses, creating a diverse and dynamic industry landscape. The strength of the private sector is further enhanced by strong partnerships between industry and academic institutions, fostering innovation and talent development.
The Dutch government and the Municipality of Amsterdam have played a crucial role in cultivating this AI ecosystem through the implementation of supportive policies. A key initiative in this regard is the Innovation Center for AI (ICAI), a national project that connects academia, companies, nonprofits, and governments to nurture the AI knowledge and talent ecosystem. ICAI's model involves the creation of dedicated research labs that focus on developing talent and knowledge in AI, providing a structured approach to advancing the field 22 . Another significant initiative is Amsterdam AI, one of seven regional hubs of the Dutch AI Coalition (NL AIC). Supported by the city municipality and the Amsterdam Economic Board, Amsterdam AI brings together universities, research institutions, and medical centers to attract investment, knowledge, and talent for AI innovations. This collaborative approach ensures that Amsterdam remains at the forefront of AI development, continuously attracting and cultivating top-tier talent. 23 24
Bengaluru, often referred to as the "Silicon Valley of India," has emerged as a global AI talent hub, ranking sixth worldwide. The city's prominence in the AI field is built upon its vibrant startup ecosystem, academic excellence, and proactive government support. Bengaluru's tech startup scene is one of the most dynamic in the world, providing a fertile ground for AI innovation and attracting talent from across India and beyond. This entrepreneurial environment is supported by the presence of leading universities and research institutes, which not only produce skilled graduates but also contribute to cutting-edge AI research. The combination of industry opportunities and academic resources creates a self-reinforcing cycle of talent attraction and development, positioning Bengaluru as a key player in the global AI landscape.
The government of Karnataka, the state where Bengaluru is located, has been instrumental in fostering the city's growth as an AI hub. 25 Recognizing the potential of AI to drive economic growth and innovation, the state government has implemented a series of policies aimed at supporting the tech sector. One of the most significant initiatives has been the focus on Global Capability Centres (GCCs). The government has introduced incentives, regulations, and policies to attract investments in AI and create digital jobs through these GCCs. Currently, Bengaluru holds a 39% market share of GCCs in India, a figure that is expected to grow with the implementation of new policies. This governmental support, combined with the city's existing strengths, ensures that Bengaluru continues to attract and develop top AI talent, solidifying its position as a global AI hub.
London stands as the second-largest European AI talent hub. The city is home to several renowned universities that offer comprehensive AI programs, producing a steady stream of skilled professionals who contribute to the local talent pool. This educational foundation is complemented by London's vibrant startup scene, which features numerous incubators, accelerators, and venture capital firms supporting AI innovation. The availability of funding and support for early-stage AI companies creates an attractive environment for entrepreneurs and researchers alike, fostering a culture of innovation and risk-taking that is essential for advancing the field of AI.
The presence of major tech companies in London further enhances its status as an AI hub. Organizations like DeepMind, Google, and Facebook have established significant AI research operations in the city, providing opportunities for high-level research and development. The UK government has also played a crucial role in supporting London's AI ecosystem through initiatives such as the AI Sector Deal, a comprehensive strategy involving financial support and policy measures 26 . This deal includes funding for AI research, reforms in technical education such as the launch of T levels, increased investment in STEM subjects, and the establishment of the Turing AI Fellowship. These government-led efforts demonstrate a commitment to developing and retaining AI talent, ensuring that London remains competitive in the global AI landscape. 27
The emergence of Amsterdam, Bengaluru, and London as prominent AI talent hubs illustrates the importance of a multifaceted approach to developing AI ecosystems. The common thread among these successful hubs seems to be the synergy between industry, academia, and government, creating environments where AI talent can thrive.
Chart 3: Comparing male vs female AI talent shares in the EU
What you see:
This chart presents the estimated male-female ratio of AI talent across various countries. On the left side, it displays the absolute number of female AI professionals in our dataset for each EU country. On the right side, it shows the percentage of the AI workforce that is female, providing a comparative view of gender distribution in AI across the EU.
Revelio Labs predicts gender based on first names using a model trained on social security administration data. If a name has a probability above 50% of being female, the individual is counted as female in this analysis. Individuals without a predicted gender (2.1% in the EU data) are omitted from this analysis. It is important to acknowledge that predicting gender based on names is not always accurate, and readers should keep this limitation of the data in mind.
What it means:
Based on our data, France, Germany, and the Netherlands have the highest absolute numbers of female AI talent in the EU. However, simply a higher number of females does not mean that the AI workforce is more gender-balanced. Latvia and Finland are among the countries with the highest female share of AI talent, exceeding 40%. Conversely, Austria, Germany, and Greece have a lower female proportion of AI professionals, close to 20%. Despite having one of the highest numbers of female AI professionals in absolute terms, Germany ranks second to last among European countries for gender balance. The size of the country might be a factor here. In absolute numbers, France has the highest number of female AI professionals, with approximately one in four AI professionals being female.
Eastern European countries generally outperform their Western European counterparts in maintaining a balanced male-female ratio within the AI workforce. Studies suggest that this higher gender parity in STEM (Science, technology, engineering and mathematics) fields in Eastern Europe can be partially attributed to the legacy of the Soviet Union, where women's participation in science was strongly encouraged 28 29 .
For instance, nearly half of Latvia's AI professionals are female, reflecting the country's labor market in STEM. Latvia has one of the highest shares of women in scientific personnel (49.6% in 2020) and is one of the few EU countries where women outnumber men in science and engineering professions, a trend also seen in Lithuania and Bulgaria 30 31 . Latvia's achievements in gender parity in the AI labor market may result from proactive policy efforts toward gender equality. In 2018, Latvia implemented the “Plan for the Promotion of Equal Rights and Opportunities for Women and Men for 2018-2020,” which was renewed for the period 2021-2023. This plan includes measures to eliminate gender stereotypes and challenge traditional gender roles 32 , which have been identified as significant factors contributing to gender gaps in STEM 33 .
The strong positioning of Finland in gender balance in AI labor markets is likely explained by its general leadership in gender equality, ranking second out of 146 countries in the World Economic Forum’s 2024 Global Gender Gap Index. This Index measures different indicators of gender parity, among them men-women balance on labor-force participation, in which Finland ranked 8th, and enrollment in secondary and tertiary education, in which Finland occupied the first position 34 . This reflects the concrete actions the Finnish government has put in place to promote gender equality. In 2022, the Government adopted a Report on Gender Equality Policy to guide Finland’s gender equality policy for this decade across different sectors and activities of society, including education and the labor market 35 .
Even if Finland does not seem to have specific legal or institutional provisions to promote gender equality in STEM or research, the country’s Act on Equality between Women and Men emphasizes the importance of preventing discrimination based on sex or gender identity and promotes gender equality, focusing on improving women’s position in employment. The Act also requires educational institutions and employers to implement plans to address gender gaps in decision-making, employment (salary, segregation, working conditions), studies, and teaching, as well as discrimination and harassment 36 . As it was pointed out, all these issues are strong determinants of women's participation and stay in STEM and AI professions, for which Finland’s frameworks to address them might be a reason behind its leadership in AI workforce gender balance.
For years, EU Members States have been raising alarms about increasing labor and skills shortages. The European Commission has identified 42 occupations which have shortages, among them are all STEM subjects 37 . To address this issue, the EC set out an action plan that establishes measures in five areas: Supporting the activation of underrepresented people in the labor market, providing support for skills development, training and education, improving working conditions in certain sectors, improving fair intra-EU mobility for workers and learners, and attracting talent from outside the EU 38 . AI labor markets could strongly benefit from these actions. For instance, appealing to the first area, the EU could provide policy support to increase the participation of women -who are currently underrepresented - in AI professions. Policy efforts can also be made to develop AI skills in EU countries and to attract foreign AI talent.
This goes in line with the priorities of the recently reelected President of the European Commission, Ursula von der Leyen. The President’s political guidelines for the next 5-year term introduce a STEM Education Strategic Plan to tackle the alarming drop in performance and the shortage of qualified teachers in science, technology, engineering, and math (STEM) fields. This strategy would also look to bring more girls and women into education and careers in STEM. Additionally, the guidelines emphasize the need to create conditions for researchers to thrive if the EU is looking to lead on innovation. This involves attracting and retaining top talent. To achieve this, von der Leyen proposed to strengthen collaboration between research departments, higher education, and business, particularly through the enhancement of University Alliances 39 .
Chart 4: AI talent's unique gender divide compared to workforce norms
What you see
These charts show the percentage of women in the general workforce of a country (orange), the percentage of women in the AI workforce of the country (lavender), and compare it with the EU average percentage of women in the AI workforce (petrol).
What it means
A gender imbalance exists in both AI labor markets and the overall workforce across all sampled countries. In most cases, this imbalance is significantly more pronounced in AI talent than in other professions. However, countries such as Latvia, Finland, Italy, Iceland, and India show a smaller gap between the general labor force and AI sector gender ratios.
While some countries like Portugal and Estonia have achieved near gender equity in their overall workforce, this balance is not reflected in their AI labor markets. For instance, in Portugal, the proportion of men and women in the general labor force is almost equal, with the gender share difference approaching zero percent. However, in the AI sector, this difference rises dramatically to 51 percent. Similar patterns are observed in Estonia and most EU Member States, where the gender imbalance in the total workforce is below 10 percent, but the disparity between men and women in AI roles ranges from 40 to 50 percent.
This stark contrast between overall workforce gender balance and AI sector imbalance persists in non-EU countries as well, with Iceland and India being notable exceptions. These findings underscore a widespread and significant gender gap in AI talent across various national contexts, even in countries that have made substantial progress towards gender equity in their broader labor markets.
Chart 5: Seniority breakdown of women in AI
What you see
These charts show the male-female ratio of AI talent across different seniority levels. The female share is in orange. We have defined the seniority based on Revelio Lab’s existing seniority metric.
The seniority metric combines information about an individual's current job (including title, company, and industry), job history (such as the duration of previous employment and the seniority of past positions), and age. These factors generate individual scores, which are then averaged to create a continuous seniority metric. To make this metric more interpretable, it is converted to an ordinal value by mapping it to recognizable seniority keywords and binning it into discrete categories. The resulting classification system consists of seven ordinal seniority levels, ranging from Entry Level to Senior Executive Level. This spectrum encompasses roles from interns and trainees at the lower end to C-suite executives at the higher end, representing a progression in responsibility and expertise throughout an individual's career path. To learn more about this breakdown, please refer to the Methodology section.
While the first chart covers the seniority of the whole AI Talent pool in our dataset, the second one focuses on the countries of the European Union.
What it means
The graph illustrates the gender disparity in AI talent, highlighting not only the imbalanced proportion of women in AI professions but also their diminishing representation at higher seniority levels. At the Entry Level, women comprise approximately 29% of AI workers, already indicating a significant gender imbalance considering that women represent half of the global population. This disparity widens as seniority increases, with women occupying less than 15% of Senior Executive roles in AI.
Multiple factors contribute to this gender imbalance in the AI workforce and the underrepresentation of women in senior positions within STEM professions. The issue originates with gender disparities in STEM education, and in many countries still, simply education. In higher education, women account for only 35% of students enrolled in STEM-related fields, and merely 28% of global researchers are female 40 . Female representation is even lower in higher-paying fields such as computer science and engineering 41 . In many countries, the primary concern is the limited educational pathways available for girls, who are significantly underrepresented in STEM subjects at the school level.
Cultural norms and gender stereotypes play a crucial role in perpetuating this imbalance. Girls are often socialized to believe that STEM fields are masculine domains and that female aptitude in STEM is inherently inferior to that of males. Despite research on biological factors dispelling these beliefs, they persist, undermining girls' confidence and interest in STEM subjects 42 . This often discourages them from pursuing these fields from a young age.
Furthermore, women continue to leave STEM disciplines at disproportionate rates during their higher education, when transitioning to the workforce, and throughout their careers 43 . This attrition partially explains the widening gender disparities in AI and STEM labor markets at higher seniority levels. Systemic discrimination, unconscious bias, and sexual harassment are among the factors that lead women to prematurely end their STEM careers 44 . For instance, unconscious biases and discriminatory practices in hiring, promotions, daily interactions, and grant funding can impede women's professional progression in STEM 45 .
Additionally, strongly male-dominated work environments, gender discrimination, the gender pay gap, and the persistent glass ceiling that impedes women from gaining expertise in high-profile emerging skills have been identified as challenges for women working in AI
46
. The work-life balance imbalance remains a significant obstacle to women's career advancement. The demanding nature of STEM careers often conflicts with family responsibilities, which disproportionately affect women due to a lack of family-friendly policies in organizational settings
47
. Women are more likely to take career breaks or reduce working hours for caregiving, leading to slower career progression.
Chart 6: Leading European AI hotspots and their gender balance
What you see
This map highlights European AI hubs, with percentages indicating female representation in each city's AI workforce. The size of the dot represents the size of the hub in terms of the number of AI professionals in the city.
What it means
This map reinforces previous discussions on the underrepresentation of women in the AI workforce across major European tech centers. The data reveals a stark contrast between thriving AI ecosystems and low female representation in leading European hubs. Notably, Milan, a relatively smaller hub, leads with 30.7% female AI professionals, while Frankfurt, Germany, has the lowest representation at just 19% female AI talent. These figures underscore the persistent gender imbalance in the AI sector, even in Europe's most advanced tech cities. The disparity appears more pronounced in larger tech hubs, suggesting that targeted initiatives to increase women's participation in AI are crucial, particularly in these major centers.
Chart 7: Origins of the foreign AI talent in the EU
What you see
This chart shows the countries of origin of foreign AI talent in the EU, US and UK. In the interactive drop-down chart, you can see where the foreign talent comes from in specific countries.
What it means
The data suggest a strong presence of talent migrating to the EU from the Global South with India leading at 12.10%, followed closely by China (11.16%). There is also significant talent inflow from the US (10.45%) but it is highly likely that these are EU nationals returning after studying/working in the US. The UK's strong presence (9.81%) post-Brexit suggests enduring ties in the AI sector. The diversity of source countries, including Iran (7.60%), Russia (4.93%), and emerging tech nations like Brazil (3.93%) and Egypt (3.29%), highlights the EU's appeal as a destination for AI professionals worldwide. This distribution reflects the EU's success in attracting talent from both established and developing tech ecosystems. The presence of countries like Pakistan (2.73%) and Argentina (2.52%) in the top 10 indicates a broad "brain gain" for the EU. The data reveals existing talent pipelines, indicating significant potential for further expansion of skilled labor immigration through strategic and forward-thinking labor market policies. Simultaneously, it's crucial to emphasize the importance of cultivating and advancing the domestic talent pool to create a balanced approach to AI workforce development.
Chart 8: Origins of the foreign female AI talent in the EU
What you see
The chart illustrates the top 10 countries of origin for female foreign AI talent in the EU.
What it means
India leads the overall AI talent influx to the EU at 12.10%, but interestingly drops to fourth place when considering only female AI talent. This suggests a gender imbalance in India's AI talent pool moving to the EU, which could be worth exploring further. China and the United States maintain strong positions in both charts, but their order is reversed for female talent, with China leading slightly for women. The UK holds a consistent third place in both scenarios, indicating a balanced gender representation in its AI talent export to the EU.
The continued strong presence of talent from countries like Iran, Brazil, and new entries like Egypt and Pakistan in this chart reinforces the significant contribution of the Global South to the EU's AI sector. This persistence across both general and female-specific talent pools suggests these countries are important sources of diverse AI expertise for the EU. This comparison not only highlights the global nature of AI talent migration but also reveals subtle gender-related patterns in this migration, which could inform targeted policies for talent attraction and diversity initiatives in the EU's tech sector.
Conclusion
This analysis of the gender gap in AI talent globally and within the European Union reveals a stark reality: The AI industry is facing a significant diversity crisis, with women severely underrepresented across all levels. Our findings highlight the urgent need for targeted interventions to increase women's participation in this rapidly growing and critically important field.
Key insights from our analysis include:
-
Women comprise only 22% of AI talent globally, a figure that drops to less than 15% at senior executive levels. This severe underrepresentation reflects and potentially exacerbates existing societal biases related to gender and race in AI systems.
-
Within the European Union, the gender disparity in AI talent varies widely. While countries like Latvia and Finland have achieved over 40% female representation in AI, major economies like Germany lag behind, with women making up only about 20% of AI professionals.
-
The gender gap in AI is significantly more pronounced than in the overall workforce. Even in countries that have achieved near gender equity in their general labor force, the AI sector shows dramatic imbalances of up to 51%.
-
Major global AI hubs, particularly in the United States, dominate the landscape of AI talent. However, even in these leading centers, female representation remains low, highlighting the pervasive nature of the gender gap.
-
Eastern European countries generally outperform their Western European counterparts in maintaining a more balanced male-female ratio within the AI workforce, possibly due to historical factors and targeted policies.
-
The EU attracts significant AI talent from the Global South, with India and China leading as sources of foreign AI professionals. This diversity in talent sources presents both opportunities and challenges for the EU's AI sector.
These findings reinforce the concerns raised in our introduction about the implications of the gender gap in AI. The stark underrepresentation of women in AI represents a significant loss of talent and potential innovation in a field that is crucial for future economic growth and technological advancement. By increasing diversity in AI development teams, we can not only reduce exclusion overhead but also enhance the overall effectiveness and applicability of AI systems across diverse user groups.
The data presented in this report provides a clear call to action for policymakers, industry leaders, and educators. As the EU aims to become a global leader in AI development and deployment, closing the gender gap must be a priority. This will not only ensure more equitable representation but also lead to the development of AI systems that work effectively and fairly for all of society. The future of AI, and its impact on Europe, depends on our ability to harness the full spectrum of human talent and perspective in its creation and implementation.
Methodology
Definition of AI talent:
We have defined AI talent in the Revelio dataset as someone who has AI skills or has/had AI employment.
Revelio Labs collects unstructured online public profiles, resumes, and job postings, and curates and structures this data through proprietary algorithms. They provided us with an initial data set of 659 million individual profiles from around the world. For each of these individuals, we have access to their educational and job history, as well as a list of their skills that were either publicly self-reported or predicted by Revelio Labs based on other profile information.
In our definition, an individual qualifies as AI talent in one of the two following ways:
-
Employment:
If any of an individual's past position titles include the words "Machine Learning," "Artificial Intelligence," “Computer Vision,” or "AI" (case insensitive for the full words), they are deemed AI talent. We also match the full terms in an English translation of foreign job titles. We omit the abbreviations in the translation, as these have different meanings in some other languages. This matching approach has two main issues:-
We disproportionately miss individuals from countries where it is uncommon to specify job titles in the ML sector in English.
-
Many people working in AI have titles like "Lead Scientist" or "Principal Engineer," which are not AI specific, thus can’t be matched
To address these problems, we also match relevant skills.
-
-
Skills:
For each user, we have a list of skills. The dataset includes 8,705 unique skills, either self-reported or predicted by Revelio's algorithms. We selected 11 skills that are sufficient to classify someone as AI talent. While many AI professionals have skills like Python or C++, these are too general to identify AI talent specifically. The selected skills, in order of frequency, are: machine learning, artificial intelligence, computer vision, natural language processing, deep learning, CUDA, neural networks, machine vision, TensorFlow, scikit-learn, and genetic algorithms. These skills mostly relate to software development in AI. While significant work is also done in hardware, those skills are too general to identify AI talent specifically. Some skills produced too many false positives: for example, robotics often included only mechanical engineering skills, NLP stood for Neuro-linguistic programming used by personal coaches, and machine translations were often listed by human translators.
Another reason we consider skill matching crucial is that AI talent and potential AI talent (students joining the workforce in the next couple of years) are not always employed in roles directly related to their expertise. Many individuals possess the necessary skills but work in positions where their AI capabilities are underutilized. By focusing on skill matching, we ensure that our dataset includes these individuals, acknowledging their potential to transition into AI roles when opportunities arise.
Matching via skills has a downside: If someone has the role of Machine Learning Engineer, we know they work extensively on AI. However, if someone lists "machine learning" as a skill, we don't know how much they use it or their level of expertise in it. In the analysis based on Revelio Labs data, each person is counted the same, regardless of how intensively they use AI skills.
From the Revelio Labs data, we identified 1,587,621 individuals as AI talent. Of that, we matched 1,007,349 individuals (63%) based solely via skills, 416,000 (26%) only via roles, and 164,272 (10%) via both skills and roles. Of the AI talent matched via skills, there is an even split between matches with predicted and self-reported skills with a 2% match via both.
The gap between profiles matched via roles and skills can be better understood through the following considerations:
-
Evolving Job Market: The AI job market is rapidly evolving, and many individuals acquire relevant skills through non-traditional pathways such as online courses, bootcamps, and self-study. These individuals may not have formal job titles reflecting their AI expertise.
-
Broad Applicability of AI Skills: AI skills are highly versatile and can be applied across various industries. As a result, many professionals with AI capabilities may hold job titles that are not explicitly AI-related but involve significant use of AI technologies.
-
Industry-Specific Roles: Certain industries may not have established AI-specific job titles, leading to talented individuals working under more general or traditional titles such as "Software Engineer," "Data Scientist," or "Research Scientist."
-
Emerging Talent: Students and recent graduates often possess up-to-date AI skills but may initially enter the workforce in roles that do not fully utilize their capabilities. As they gain experience, they are likely to transition into more specialized AI roles.
-
Self-Reporting of Skills: Cultural differences in self-reporting skills exist across various regions and countries. Additionally, individuals with job titles that clearly indicate they work in AI-related roles might not feel compelled to list their skills on their profiles. This is where predicted skills become particularly useful, as they help bridge the gap and provide a more comprehensive view of an individual's capabilities, even when not explicitly listed.
-
Internal Company Structures: Companies may not always update job titles to reflect the evolving skill sets of their employees, especially in large organizations with rigid job title frameworks. Employees may develop AI expertise without a corresponding change in their job title.
Despite the inherent challenges in defining and identifying AI talent, our combined approach of matching both job titles and skills provides a robust and comprehensive method for approximating the global AI workforce. While no methodology is without limitations, we believe that this dual-pronged strategy, leveraging Revelio's extensive dataset and predictive algorithms, offers a comparatively accurate representation of the AI talent landscape. This approach not only captures professionals in explicitly AI-related roles but also identifies individuals with relevant skills who may be working in adjacent or non-traditional AI positions. As such, it provides a solid foundation for analyzing trends, disparities, and opportunities in the global AI talent pool, making it a valuable tool for policymakers, educators, and industry leaders seeking to address the challenges and harness the potential of AI workforce development.
Data Coverage and Quality Tests:
To check data coverage in the Revelio Labs dataset, we compute the share of each country's labor force included in our dataset using 2023 labor force estimates from the World Bank 48 . For example, the US and the Netherlands have coverage rates of 94% and 89%, respectively. In contrast, Germany has a coverage rate of only 25%, the 5th lowest in the EU. This lower coverage could be due to stronger privacy concerns in Germany, as Revelio only uses publicly accessible data. Detailed numbers can be found in the following table:
Country Name |
Number of Individuals in Revelio Data |
Labor Force |
Share of Labor Force in Revelio Data |
---|---|---|---|
United States |
160674372 |
170602911 |
94.18 |
Netherlands |
8845651 |
9925443 |
89.12 |
Canada |
17624569 |
21639790 |
81.45 |
United Kingdom |
27709598 |
34673438 |
79.92 |
Denmark |
2508246 |
3169686 |
79.13 |
Malta |
236881 |
301690 |
78.52 |
Australia |
11189910 |
14293694 |
78.29 |
Luxembourg |
267832 |
347323 |
77.11 |
Ireland |
2085378 |
2712772 |
76.87 |
Iceland |
164549 |
233608 |
70.44 |
France |
20418528 |
31790395 |
64.23 |
Switzerland |
3197163 |
4978943 |
64.21 |
Norway |
1867277 |
2996483 |
62.32 |
New Zealand |
1884601 |
3033434 |
62.13 |
Sweden |
3522240 |
5829755 |
60.42 |
Portugal |
2910904 |
5345459 |
54.46 |
Belgium |
2908390 |
5382321 |
54.04 |
Italy |
13553049 |
25661937 |
52.81 |
Spain |
12107644 |
23788645 |
50.9 |
Chile |
4889324 |
9847754 |
49.65 |
Finland |
1331182 |
2866601 |
46.44 |
Cyprus |
298881 |
692875 |
43.14 |
Brazil |
43160380 |
108695239 |
39.71 |
Israel |
1649324 |
4527473 |
36.43 |
Croatia |
582297 |
1735594 |
33.55 |
South Africa |
8030077 |
25158403 |
31.92 |
Greece |
1484242 |
4655750 |
31.88 |
Austria |
1492660 |
4784783 |
31.2 |
Romania |
2531671 |
8134075 |
31.12 |
Latvia |
295233 |
965500 |
30.58 |
Estonia |
223408 |
740661 |
30.16 |
Slovenia |
315068 |
1050575 |
29.99 |
Czech Republic |
1609777 |
5404325 |
29.79 |
Colombia |
7653510 |
26003385 |
29.43 |
Lithuania |
433049 |
1474043 |
29.38 |
Turkey |
9820041 |
35111887 |
27.97 |
Germany |
11097161 |
44368658 |
25.01 |
Bulgaria |
723548 |
3081382 |
23.48 |
Poland |
4085395 |
17986215 |
22.71 |
Mexico |
13398135 |
60042318 |
22.31 |
Hungary |
958568 |
4936209 |
19.42 |
Philippines |
8306496 |
49477060 |
16.79 |
Egypt |
4856997 |
33430910 |
14.53 |
India |
68019395 |
593729164 |
11.46 |
Indonesia |
15659759 |
140931083 |
11.11 |
Iran |
2907905 |
29158831 |
9.97 |
Kenya |
2455929 |
25501846 |
9.63 |
South Korea |
2251947 |
29523205 |
7.63 |
Pakistan |
5773435 |
80989797 |
7.13 |
Nigeria |
5374645 |
75721345 |
7.1 |
Russia |
4712868 |
72352166 |
6.51 |
Thailand |
2338719 |
40813511 |
5.73 |
Bangladesh |
3038557 |
74913612 |
4.06 |
China |
30150587 |
779890786 |
3.87 |
Japan |
2376627 |
69278589 |
3.43 |
Myanmar |
438015 |
22884069 |
1.91 |
Ethiopia |
561021 |
61664369 |
0.91 |
We are wary of using data for countries that have lower coverage based on the chart above. It is important to note, however, that the talent pool we are exploring in this study is assumed to have access to the internet, job portals, and basic digital literacy to self-report skills on online platforms. In India, for example, only 11.46% of the labor force is represented in the Revelio Labs data. We assume, however, that most AI talent is represented in this pool to make our analysis valid.
Accuracy:
We tested our matching algorithm for precision by randomly selecting a sample of 100 people to check whether they truly qualify as AI talent by visiting their online career platform profiles. In this test, we were not able to confirm 16% of the sample. This was to be expected because some of the information in the dataset could have been removed from users’ profiles, while some other users could have deleted their profiles altogether or have changed their profile URL. Testing recall is much more challenging since AI talent makes up only a small fraction of global online career platform members. As mentioned previously, our data may miss individuals due to various reasons, such as unspecific role titles or a lack of self-reported skills.
General Limitations
Using Undergraduate Degrees as Origin Proxies
The country of an individual’s undergraduate degree serves as a proxy for their origin, based on the assumption that most people pursue their early education in their country of origin. This method is generally reliable; for instance, OECD data 49 cases. Nonetheless, we acknowledge outliers like Australia and New Zealand, where the share of international undergraduates peaks at 28%, which could be due to English being their official language. Further evidence supports this hypothesis: the UK (18%) and Austria (17%) have high percentages of international undergraduates, contrasting with lower percentages in Southern European countries like Italy (4%) and Spain (2%). A case that challenges this hypothesis is the United States, where, despite the English language being the medium of education, only 4% of students at the undergraduate level are international students. For detailed statistics, please refer to the OECD report "Education at a Glance 2020." 50 Not all countries of origin in our dataset are OECD countries. In our analysis, we verified a low percentage of international students from the most relevant countries. In India, for example, the percentage of international students at the undergraduate level is less than 0.5%. 51
When interpreting our findings, we take into account the variances in international student percentages across different countries. These variances can influence the perceived origin of AI talent. For example, our analysis may reveal that a percentage of AI professionals working in Ireland received their degrees in the UK. Given the UK's high proportion of international students (18%), a fraction of this group might actually originate from other countries. We believe that these effects do not substantially alter the primary insights regarding key source countries for AI talent.
Gender prediction Limitation
While the data does not feature ground truth gender information, Revelio Labs estimates users' gender using census data information: each user's first name is checked against national census registries to estimate the gender shares of people with that name. This method can present issues with names that are not common: for those, the gender assignment is essentially random; nonetheless, they are by distribution a small minority of the population.
A significant limitation in our dataset is the binary representation of gender (male and female), which fails to capture the diverse spectrum of gender identities present in the workforce.
Given the scope and scale of our study, and the limitations of available global data sources, this binary representation is unavoidable for achieving consistent analysis across different regions and demographics. However, we recognize the importance of more inclusive gender representations and advocate for future improvements in data collection methods that can better capture the full spectrum of gender identities in the workforce.
Self-Reporting
Both datasets primarily rely on self-reported information from users of professional career networks. This approach has two inherent limitations: individuals may either not be present on a given career network or may use the network but provide incomplete or inaccurate information. In Chart 2, for example, we only see LinkedIn members who have provided a Bachelor’s degree on LinkedIn. These limitations can introduce selection effects that skew our results. Even though we select countries with large coverage, the likelihood of having a profile can vary based on the country of origin or the highest degrees attained. For example, Chinese professionals working on AI in France might be undercounted if they are less likely to create profiles than French nationals in France.
Furthermore, individuals from different backgrounds may have varying habits regarding skill self-reporting, which can affect the identification of AI talent. Despite these limitations, LinkedIn, with over 1 billion members globally, offers the most comprehensive global dataset on career paths to analyze AI talent flows in Europe and beyond.
The Revelio Labs dataset partially addresses the lack of self-reporting by including predicted skills. They also account for geographical differences in profile creation by applying sampling weights to adjust for roles and locations underrepresented in the sample (as described before). This approach helps approximate the true estimate of the underlying population as closely as possible.
This limitation is further mitigated in this paper's context, as we are mainly examining a more technologically advanced segment of the global workforce. These individuals typically possess the digital literacy and necessary equipment to create and maintain profiles on professional career websites. They often bear the benefits of self-reporting their skills.
Skill Taxonomy Limitations
The current skill taxonomy used by Revelio may not encompass all the necessary skills for specific AI domains, such as AI chip development. This gap means that professionals with specialized skills in areas like hardware or AI chip design might not be adequately recognized or included in the dataset. The absence of these specialized skills in the taxonomy can skew the understanding of the full spectrum of AI talent and hinder the comprehensive identification of expertise across all AI-related fields.
This paper is being published as part of the project 'Strength and weaknesses of the German and European AI ecosystem - talent in the focus'. The project is funded by the Carl Zeiss Stiftung.
1 www.weforum.org/publications/global-gender-gap-report-2024/
2 https://ainowinstitute.org/publication/discriminating-systems-gender-race-and-power-in-ai-2
3 https://dl.acm.org/doi/abs/10.1145/3531146.3533114
4 https://time.com/5520558/artificial-intelligence-racial-gender-bias/
5 https://www.human-technology-foundation.org/news/diversity-in-ai-towards-a-problem-statement
6 https://www.nature.com/articles/d41586-023-01689-4
7 https://www.electronicspecifier.com/products/artificial-intelligence/the-ai-industry-is-suffering-from-a-diversity-crisis
8 https://www.autodesk.com/design-make/articles/diversity-in-ai
9 https://time.com/5520558/artificial-intelligence-racial-gender-bias/
10 https://cset.georgetown.edu/wp-content/uploads/CSET-Examining-Singapores-AI-Progress.pdf
11 https://www.smartnation.gov.sg/nais/
12 https://www.cnbc.com/2023/12/05/singapore-to-triple-ai-talent-to-15000-experts-deputy-pm-says.html
13 https://www.channelnewsasia.com/singapore/national-ai-strategy-artificial-intelligence-talent-iconic-site-3963971
14 https://petir.sg/2024/03/03/singapore-plans-to-build-an-ai-talent-pool-8-key-takeaways-on-governments-efforts-build-a-smart-nation/
15 https://www.oecd-ilibrary.org/education/oecd-skills-strategy-luxembourg_4b9300d7-en
16 https://www.stiftung-nv.de/publications/where-is-europes-ai-workforce-coming-from
17 https://www.oecd-ilibrary.org/education/oecd-skills-strategy-luxembourg_4b9300d7-en
18 https://innovative-initiatives.public.lu/stories/luxembourgs-strategic-vision-ai
19 https://www.whitehouse.gov/briefing-room/presidential-actions/2023/10/30/executive-order-on-the-safe-secure-and-trustworthy-development-and-use-of-artificial-intelligence/
20 https://carnegieendowment.org/research/2024/01/the-silicon-valley-model-and-technological-trajectories-in-context?lang=en
21 https://carnegieendowment.org/research/2024/01/the-silicon-valley-model-and-technological-trajectories-in-context?lang=en
23 https://www.amsterdamai.com/en/nieuws/1fdba4af-bcf5-2927-a618-e1bdec02c283/Amsterdam-AI-versterkt-samenwerking-met-nieuwe-overeenkomst
24 https://www.amsterdamai.com/en/about
25 https://thesouthfirst.com/karnataka/bengaluru-emerges-as-worlds-second-largest-ai-talent-pool-solidifying-its-global-hub-status/
26 https://www.gov.uk/government/publications/artificial-intelligence-sector-deal/ai-sector-deal
27 https://www.gov.uk/government/publications/turing-artificial-intelligence-fellowships/turing-artificial-intelligence-fellowships
28 https://www.weforum.org/agenda/2019/03/gender-equality-in-stem-is-possible/
29 https://www.worldbank.org/en/topic/gender/publication/the-equality-equation-advancing-the-participation-of-women-and-girls-in-stem
30 https://www.unwomen.org/sites/default/files/2022-06/Latvia%20%28EN%29.pdf
31 https://www.weforum.org/agenda/2019/03/gender-equality-in-stem-is-possible/
32 https://www.unwomen.org/sites/default/files/2022-06/Latvia%20%28EN%29.pdf
33 https://www.worldbank.org/en/topic/gender/publication/the-equality-equation-advancing-the-participation-of-women-and-girls-in-stem
34 https://www.weforum.org/publications/global-gender-gap-report-2024/
35 https://valtioneuvosto.fi/en/-/1271139/the-government-adopted-a-report-on-gender-equality-policy-on-9-june-2022.-the-report-outlines-finland-s-national-and-international-gender-equality-policy-until-the-end-of-the-2020s
36 https://eige.europa.eu/gender-mainstreaming/toolkits/gear/legislative-policy-backgrounds/finland?language_content_entity=en
37 https://eur-lex.europa.eu/legal-content/EN/TXT/?uri=COM%3A2023%3A716%3AFIN
38 https://ec.europa.eu/commission/presscorner/detail/en/ip_24_1507
39 https://commission.europa.eu/document/download/e6cd4328-673c-4e7a-8683-f63ffb2cf648_en?filename=Political%20Guidelines%202024-2029_EN.pdf
40 https://www.unesco.org/en/articles/new-unesco-report-sheds-light-gender-inequality-stem-education
41 https://www.aauw.org/resources/research/the-stem-gap/
42 https://www.unesco.org/en/articles/new-unesco-report-sheds-light-gender-inequality-stem-education
43 https://www.unesco.org/en/articles/new-unesco-report-sheds-light-gender-inequality-stem-education
44 https://professionalprograms.mit.edu/blog/leadership/the-gender-gap-in-stem/
45 https://professionalprograms.mit.edu/blog/leadership/the-gender-gap-in-stem/
46 https://eige.europa.eu/publications-resources/publications/artificial-intelligence-platform-work-and-gender-equality
47 https://professionalprograms.mit.edu/blog/leadership/the-gender-gap-in-stem/
48 https://data.worldbank.org/indicator/SL.TLF.TOTL.IN
49 https://www.oecd-ilibrary.org/education/education-at-a-glance-2020_69096873-en
50 https://www.oecd-ilibrary.org/education/education-at-a-glance-2020_69096873-en
51 https://brill.com/display/book/9789463511612/BP000055.xml
Authors
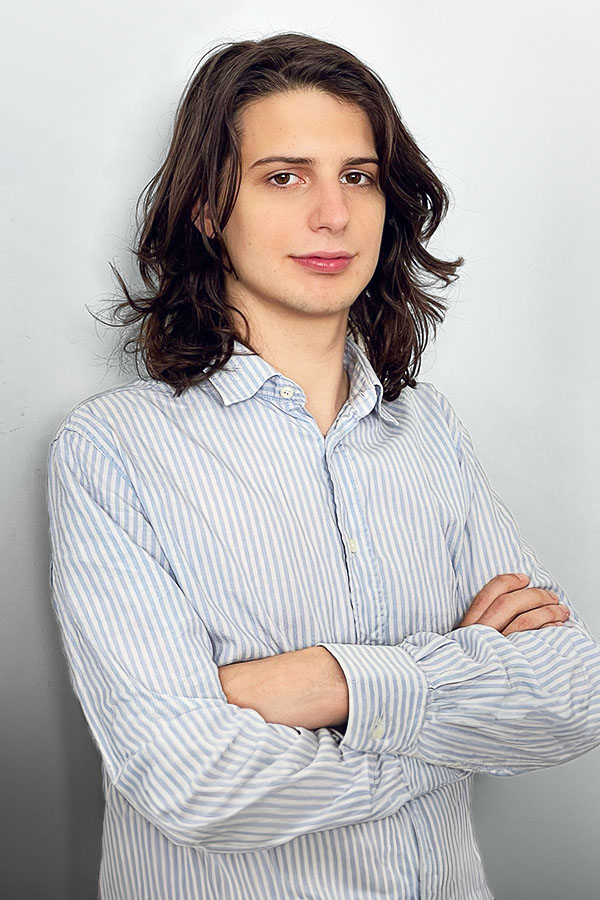
Ruggero Marino Lazzaroni
Data Scientist
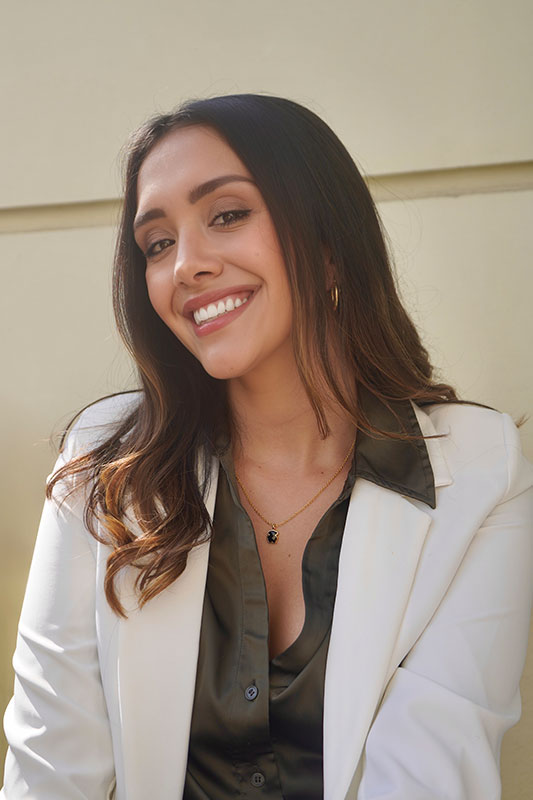
Paula Mendoza
Summer Researcher